Essays (subscribe)
- I Eat Patience for Breakfast
- Asking for Compensation Strategy Changes
- Inside the Cue COVID-19 Test Cartridge
- Space Advocacy
- Preparing a Virtual Conference Talk
- On My Way To Space
- A tale about a Legacy Application and Infrastructure as Code
- Visiting the Duga-1 Radar in the Chernobyl Exclusion Zone
- My Path to Site Reliability Management
- Magic Mail from David Copperfield
- Flash Cards for Site Reliability Engineers
- IP over Web-avian Carriers
- The Machine That Hires Me
- How To Write A Tiny Shell In C
- UNIX-like processes and the imaginary disco ball
- About Shell And Echo
- Replying To Domain Abuse Mail
- A Concurrency-safe, Iterable Binary Search Tree
- Go Contain Me
- IPv6 Workshop
- Looping AIs (Siri, Alexa, Google Home)
- Building an encrypted travel wifi router
Bio
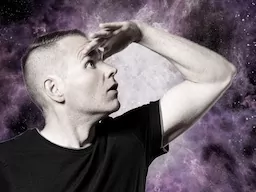
Dan Luedtke is an engineering leader who served his country, worked as IT security consultant, wrote a book about IPv6, built an autonomous system from scratch and dreams of space travel. Dan has lived in Munich, London and the San Fracisco Bay Area. As a Site Reliability Engineer Dan operates Google's global network load balancer Maglev. Outside of work he is on a mission to build an off-grid, low energy house in the Santa Cruz mountains. Dan has been awarded the noble title Duke of Principality of Sealand in exchange for a domain name. Dan's one time attempt at making art went viral.
Talks
-
Implementing Distributed
Consensus
Virtual SREcon20 Americas; Dec 2020 -
Implementing
Distributed Consensus (Workshop)
SREcon19 EMEA, Dublin, Ireland; Oct 2019 -
Magic Mail
Write The Docs, Prague, Czech Republic; Sep 2019
-
Implementing Distributed
Consensus
SREcon19 Asia/Pacific, Singapore; Jun 2019 -
IP over Web-Avian Carriers (IPoWAC)
35C3, Leipzig, Germany; Dec 2018 -
Implementing
Distributed Consensus
Site Reliability Engineering Meetup, Munich, Germany; Dec 2018 -
How we un-scattered our DNS setup
and unlocked new automation options
SREcon18 Europe, Düsseldorf, Germany; Aug 2018 -
SREMUC Launch Event
and SREcon18 Americas Recap
Site Reliability Engineering Meetup, Munich, Germany; May 2018 -
Human-friendly DNS powered by
Golang
Gophers Meetup, Munich, Germany; Aug 2017 -
Design Jam, Holo Lense Prototype
“QRreconstruct”
Design Jam Meetup, Munich, Germany; Apr 2017 -
Founder Institute Survival
Guide
Founder Meetup, Munich, Germany; Mar 2016 -
sixfw - thinking ipv6 first
32C3, Hamburg, Germany; Dec 2015 -
ratools 2014 field
test
V6 World Congress, Paris, France; Mar 2015 -
ratools and NAT64 on the 31C3
congress network
31C3, Hamburg, Germany; Dec 2014 -
Introducing ratools for
Linux
IPv6-Kongress, Frankfurt (Main), Germany; May 2014 -
The Lanyard Filesystem
29C3, Hamburg, Germany; Dec 2012 -
IPv6 an der UniBwM
(German)
IPv6-Kongress, Frankfurt (Main), Germany; May 2012 -
SSL/TLS and the BEAST attack
UniBw, Munich, Germany; Apr 2012 -
Oops! Webshop Hacking
UniBw, Munich, Germany; Jan 2012